Meta AI open source的問題,透過圖書和論文來找解法和答案更準確安心。 我們找到附近那裡買和營業時間的推薦產品
Meta AI open source的問題,我們搜遍了碩博士論文和台灣出版的書籍,推薦寫的 Deep Reinforcement Learning: Fundamentals, Research and Applications 和的 Deep Reinforcement Learning: Fundamentals, Research and Applications都 可以從中找到所需的評價。
這兩本書分別來自 和所出版 。
國立臺灣科技大學 資訊管理系 盛麗慧所指導 SAIDE的 新興科技時代B2B背景下的行銷數位化創新:擴散創新視角與激進和漸進創新的中介效應 (2021),提出Meta AI open source關鍵因素是什麼,來自於行銷數乏化創新、創新傳播理論、激進創新、漸進式創新、IT探索、IT開發、B2B背景。
而第二篇論文國立雲林科技大學 工業工程與管理系 侯東旭所指導 曾莉萍的 內外科護理學與重症護理課程導入臨床模擬情境與資訊科技融入教學之成效 (2021),提出因為有 模擬情境、客觀結構式臨床測驗、資訊科技融入教學、情境知覺的重點而找出了 Meta AI open source的解答。
Deep Reinforcement Learning: Fundamentals, Research and Applications
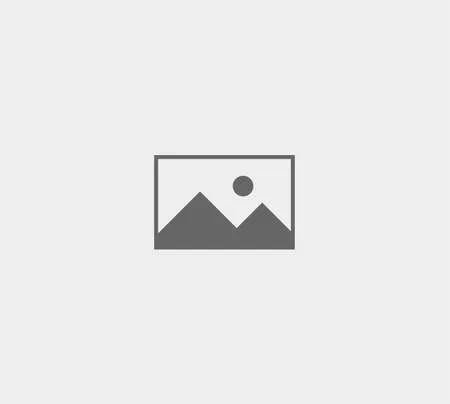
為了解決Meta AI open source 的問題,作者 這樣論述:
Dr. Hao Dong is currently an Assistant Professor at Peking University. He received his Ph.D. in Computing from Imperial College London in 2019, supervised by Prof. Yike Guo. Hao’s research chiefly involves Deep Learning and Computer Vision, with the goal of reducing the amount of data required for l
earning intelligent systems. He is passionate about popularizing artificial intelligence technologies and established TensorLayer, a deep learning and reinforcement learning library for scientists and engineers, which won the Best Open Source Software Award at ACM Multimedia 2017.Zihan Ding received
his M.Sc. degree in Machine Learning with distinction from the Department of Computing, Imperial College London, supervised by Dr. Edward Johns. He holds double Bachelor degrees from the University of Science and Technology of China: in Photoelectric Information Science and Engineering (Physics) an
d in Computer Science and Technology. His research interests include deep reinforcement learning, robotics, computer vision, quantum computation and machine learning. He has published papers in ICRA, AAAI, NIPS, IJCAI, and Physical Review. He also contributed to the open-source projects TensorLayer
RLzoo, TensorLet and Arena.Dr. Shanghang Zhang is a postdoctoral research fellow in the Berkeley AI Research (BAIR) Lab, the Department of Electrical Engineering and Computer Sciences, UC Berkeley, USA. She received her Ph.D. from Carnegie Mellon University in 2018. Her research interests cover deep
learning, computer vision, and reinforcement learning, as reflected in her numerous publications in top-tier journals and conference proceedings, including NeurIPS, CVPR, ICCV, and AAAI. Her research mainly focuses on machine learning with limited training data, including low-shot learning, domain
adaptation, and meta-learning, which enables the learning system to automatically adapt to real-world variations and new environments. She was one of the "2018 Rising Stars in EECS" (a highly selective program launched at MIT in 2012, which has since been hosted at UC Berkeley, Carnegie Mellon, and
Stanford annually). She has also been selected for the Adobe Academic Collaboration Fund, Qualcomm Innovation Fellowship (QInF) Finalist Award, and Chiang Chen Overseas Graduate Fellowship.
新興科技時代B2B背景下的行銷數位化創新:擴散創新視角與激進和漸進創新的中介效應
為了解決Meta AI open source 的問題,作者SAIDE 這樣論述:
TABLE OF CONTENTS摘要 iABSTRACT iiACKNOWLEDGEMENT iiiTABLE OF CONTENTS ivLIST OF FIGURES viLIST OF TABLES vii1. INTRODUCTION 11.1. Background and Research Motivations 11.2. Research Gaps 81.3. Research Questions and Purpose of the Study 111.4. Organization
of the Dissertation 142. LITERATURE REVIEW 152.1. Theoretical Background 152.1.1. Marketing Digitalization Innovation 152.1.2. Diffusion of Innovations Theory (DOI) 162.1.3. IT Exploration 172.1.4. IT Exploitation 192.1.5. Radical Innovation 202.1.6.
Incremental Innovation 212.2. Hypothesis Formulation 222.2.1. IT Exploration, IT Exploitation, Radical Innovation, Incremental Innovation, and Marketing Digitalization Innovation 232.2.2. The Mediating Roles of Radical Innovation and Incremental Innovation 283. RESEARCH METHO
DOLOGY 323.1. Respondents and Survey Mechanism 323.2. Measures and Survey Instrument 343.3. Control Instruments 363.4. Marker Instrument 374. RESULTS 384.1. Construct Assessment 384.2. Structural Model Evaluation 424.3. Direct Effects 434.4. Medi
ation Effects 435. DISCUSSIONS 455.1. Theoretical and Research Contributions 465.2. Managerial and Practical Contributions 515.3. Dissertation Limitation 615.4. Future Research 636. REFERENCES 64APPENDIX 80Questionnaire Survey 80
Deep Reinforcement Learning: Fundamentals, Research and Applications
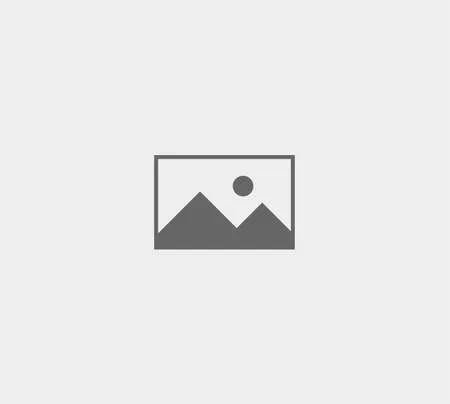
為了解決Meta AI open source 的問題,作者 這樣論述:
Dr. Hao Dong is currently an Assistant Professor at Peking University. He received his Ph.D. in Computing from Imperial College London in 2019, supervised by Prof. Yike Guo. Hao’s research chiefly involves Deep Learning and Computer Vision, with the goal of reducing the amount of data required for l
earning intelligent systems. He is passionate about popularizing artificial intelligence technologies and established TensorLayer, a deep learning and reinforcement learning library for scientists and engineers, which won the Best Open Source Software Award at ACM Multimedia 2017.Zihan Ding received
his M.Sc. degree in Machine Learning with distinction from the Department of Computing, Imperial College London, supervised by Dr. Edward Johns. He holds double Bachelor degrees from the University of Science and Technology of China: in Photoelectric Information Science and Engineering (Physics) an
d in Computer Science and Technology. His research interests include deep reinforcement learning, robotics, computer vision, quantum computation and machine learning. He has published papers in ICRA, AAAI, NIPS, IJCAI, and Physical Review. He also contributed to the open-source projects TensorLayer
RLzoo, TensorLet and Arena.Dr. Shanghang Zhang is a postdoctoral research fellow in the Berkeley AI Research (BAIR) Lab, the Department of Electrical Engineering and Computer Sciences, UC Berkeley, USA. She received her Ph.D. from Carnegie Mellon University in 2018. Her research interests cover deep
learning, computer vision, and reinforcement learning, as reflected in her numerous publications in top-tier journals and conference proceedings, including NeurIPS, CVPR, ICCV, and AAAI. Her research mainly focuses on machine learning with limited training data, including low-shot learning, domain
adaptation, and meta-learning, which enables the learning system to automatically adapt to real-world variations and new environments. She was one of the "2018 Rising Stars in EECS" (a highly selective program launched at MIT in 2012, which has since been hosted at UC Berkeley, Carnegie Mellon, and
Stanford annually). She has also been selected for the Adobe Academic Collaboration Fund, Qualcomm Innovation Fellowship (QInF) Finalist Award, and Chiang Chen Overseas Graduate Fellowship.
內外科護理學與重症護理課程導入臨床模擬情境與資訊科技融入教學之成效
為了解決Meta AI open source 的問題,作者曾莉萍 這樣論述:
護理為高度的實務作業,而客觀結構式臨床測驗(Objective Structured ClinicalExamination,OSCE)是模擬實務情境有效的臨床能力評估方法。護理人員具備良好情境知覺(Situation Awareness, SA)對於病人安全及照護結果至關重要。本研究針對南臺灣某醫護專科學校內外科及重症護理學課程,探討其導入臨床模擬情境及資訊科技融入教學之創新教學成效。 本研究為立意取樣,共收案專四護生 120 人(實驗組 61 人,對照組 59 人),專五護生 65 人。以 ADDIE 模型建置五項臨床模擬情境教案,以急性心肌梗塞病人經皮冠狀動脈氣球擴張術及支架置放護理、
基本救命術與自動體外電擊操作、硬腦膜下出血護理等主題發展 OSCE 評分表,內容效度均達 0.981 以上,IRR 具高度一致性。OSCE 成績可作為學科總結性評量之效標。經獨立樣本 t 檢定,專四實驗組與對照組其三年級學科總結性評量未達顯著差異,而四年級的學科總結性評量及內外科護理實驗成績均呈現統計顯著,顯示導入創新教學及傳統教學對於護生學科成績有差異。皮爾森相關分析發現,實驗組及對照組內外科臨床實習成績並無顯著,顯示兩項教學方式對於護生臨床實習無相關。獨立樣本 t 檢定中,兩組OSCE 呈現顯著差異,顯示導入教學方式影響 OSCE 成形性評量。比較專四與專五學生修正式 OSCE 總成績,顯
示導入創新教學對於兩組 OSCE 成形性評量有差異。OSCE 主題 SA1、SA2 及 SA3 呈現顯著,顯示導入創新教學對於專五與專四護生情境知覺有差異。專四及專五學生對創新教學滿意度高且未有差異。 本研究結果發現創新教學有助於提升學生表現,達較高水準之 SA,科技融入教學及修正式 OSCE 的應用可優化情境教學。未來建議採單一變項設計模擬教案,OSCE 搭配學程開發並延長介入,考量以學習者為中心之難度設計與 SA 各水準之分配,並強化 SA 訓練及推展於學校教育、實習及臨床,以達護理專業職涯發展。