Reenactment的問題,我們搜遍了碩博士論文和台灣出版的書籍,推薦Gwynne, John寫的 The Hunger of the Gods 和Reagin, Nancy的 Re-Living the American Frontier: Western Fandoms, Reenactment, and Historical Hobbyists in Germany and America Since 1900都 可以從中找到所需的評價。
另外網站The Crossing Reenactment | Washington Crossing Historic Park也說明:Each December, thousands of people gather on the banks of the Delaware River to watch the reenactment of George Washington's daring 1776 Christmas night ...
這兩本書分別來自 和所出版 。
國立雲林科技大學 資訊管理系 古東明所指導 吳靜瑜的 深度偽造語音之辨識檢測 (2021),提出Reenactment關鍵因素是什麼,來自於表徵學習、轉移學習、自然語言處理、深度偽造。
而第二篇論文國立臺灣科技大學 資訊工程系 陳怡伶所指導 Joshua C. Manzano的 Facestamp: Self-Reference Proactive Deepfake Detection using Facial Attribute Deep Watermarking (2021),提出因為有 的重點而找出了 Reenactment的解答。
最後網站Annual Live Twitter Reenactment | Milwaukee Public Museum則補充:MPM's Education Department, supported by community volunteers and local history graduate students, create an annual reenactment on Twitter of an event from ...
The Hunger of the Gods
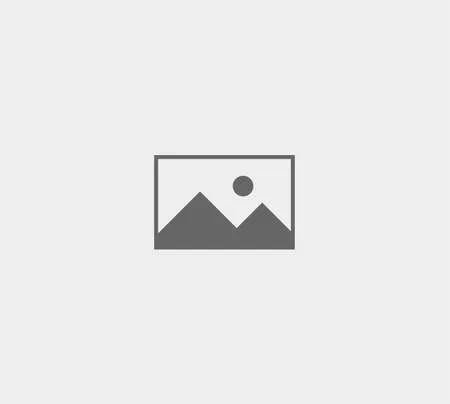
為了解決Reenactment 的問題,作者Gwynne, John 這樣論述:
John Gwynne studied and lectured at Brighton University. He’s played double bass in a rock ’n’ roll band and traveled the USA and Canada. He is married with four children and lives in Eastbourne, where he is part of a Viking reenactment group. When not writing, he can often be found standing in a sh
ield wall with his three sons about him. His dogs think he is their slave.
Reenactment進入發燒排行的影片
VLOG 138/2016: We ditched the script and adlib-ed all the way! Azmi is excited to meet his idol Freddie upclose and personal as we see in his reenactment. Thank you Freddie for being such a sport
深度偽造語音之辨識檢測
為了解決Reenactment 的問題,作者吳靜瑜 這樣論述:
摘要 iAbstract ii目錄 iii表目錄 v圖目錄 vi壹、 緒論 11.1 研究背景 11.2 研究動機 21.3 研究目的 31.4 研究架構 4貳、 文獻探討 52.1 人工智慧(Artificial intelligence) 52.1.1 機器學習(Machine Learning) 52.1.2 深度學習(Deep Learning) 52.2 語音識別 62.2.1 語音識別流程 62.2.2 聲學特徵 72.2.3 線性預估倒頻譜係數(LPCC) 72.2.4 梅爾頻率倒譜係數(MFCCs) 82.2.
5 MFCC計算步驟 92.3 語者驗證 122.4 x-vector 122.5 相關研究 132.5.1 變聲器原理 132.5.2 語音合成 142.5.3 Clone voice 152.5.4 深度偽造技術 162.5.5 深度偽造技術介紹 172.5.6 深度偽造技術應用 192.5.7 深度偽造技術現況 19參、 研究方法 223.1 研究架構 223.2 系統模組化 233.2.1 語音獲取與實驗設備 253.3 實驗流程 253.4 辨識系統 263.4.1 資料集介紹 283.4.2 預處理 293
.4.3 特徵擷取 293.4.4 X-vector 303.4.5 模型評估 333.4.6 激活函數 343.5 聲紋系統 353.5.1 資料集介紹 353.5.2 特徵擷取 363.5.3 GMM 403.5.4 語者註冊與驗證 403.5.5 模型評估 41肆、 實驗結果 424.1 辨識系統實驗結果 424.2 聲紋系統實驗結果 43伍、 結論 525.1 結論 525.2 研究限制及未來展望 52參考文獻 53
Re-Living the American Frontier: Western Fandoms, Reenactment, and Historical Hobbyists in Germany and America Since 1900
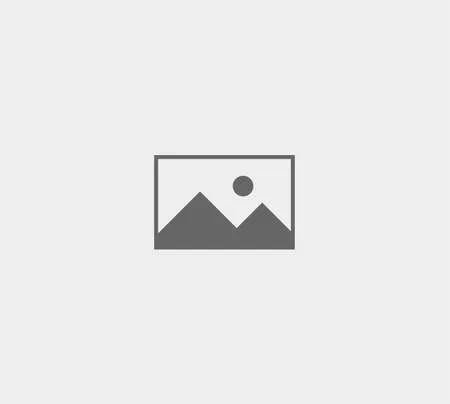
為了解決Reenactment 的問題,作者Reagin, Nancy 這樣論述:
Nancy Reagin is professor of European history and gender studies at Pace University in New York City. She is author of A German Women’s Movement: Class and Gender in Hanover, 1880-1933. She lives in Montclair, New Jersey.
Facestamp: Self-Reference Proactive Deepfake Detection using Facial Attribute Deep Watermarking
為了解決Reenactment 的問題,作者Joshua C. Manzano 這樣論述:
Deepfakes are progressively harder to distinguish and present a growingproblem to image authenticity in society. Existing studies that focus ondeepfake detection rely on artifacts or flaws generated by the deepfakeprocess which may not be present in novel deepfake models. This necessitates a proact
ive approach that is more robust and generalizable. Recentworks on proactive defense rely on deep watermarking, where they embed a Unique Identification (UID) to an image. To verify authenticity, atrusted authority needs to decode its hidden UID and cross-reference it to acentralized dataset contain
ing all existing UIDs. Overall, its reliance on atrusted centralized authority that stores individual UIDs makes it inflexibleand impedes its widespread adoption. Moreover, this authentication approach has constrained effectiveness when the number of users is limited.In this paper, we present Facest
amp, a deep watermarking model for a self-reference proactive defense against deepfakes. We address this problemby directly embedding facial attributes, instead of a UID, to an image using deep watermarking. Image-derived attributes such as facial attributesverify the legitimacy of the image through
the identification of inconsistencies between the decoded attributes and current attributes present in theimage. This eliminates the need for a centralized verification process andenables independent verification. In our experiments, we show that Facestamp allows the recovery of facial attributes i
n the wild and the subsequentverification of the current face to determine the legitimacy of the givenimage. Facestamp is able to defend against deepfakes across three deepfake models, showing promising performance in two popular datasets andis more robust to common post-processing image operations
compared to existing methods.
想知道Reenactment更多一定要看下面主題
Reenactment的網路口碑排行榜
-
#1.re-enactment中文(繁體)翻譯:劍橋詞典
re-enactment翻譯:(對事件的)再現,重現。了解更多。 於 dictionary.cambridge.org -
#2.reenactment | The Texas Military Force Museum
The Texas Military Forces museum presents “Over There-1918” A Living History program of the “Great War” experience of the US soldier. 於 www.texasmilitaryforcesmuseum.org -
#3.The Crossing Reenactment | Washington Crossing Historic Park
Each December, thousands of people gather on the banks of the Delaware River to watch the reenactment of George Washington's daring 1776 Christmas night ... 於 www.washingtoncrossingpark.org -
#4.Annual Live Twitter Reenactment | Milwaukee Public Museum
MPM's Education Department, supported by community volunteers and local history graduate students, create an annual reenactment on Twitter of an event from ... 於 www.mpm.edu -
#5.Reenactment Images, Stock Photos & Vectors | Shutterstock
Find reenactment stock images in HD and millions of other royalty-free stock photos, illustrations and vectors in the Shutterstock collection. 於 www.shutterstock.com -
#6.Battle of Honey Springs Reenactments - Oklahoma Historical ...
The next reenactment will be held in 2023. This biennial event includes two battle reenactments and self-guided tours through the Union, Confederate, and ... 於 www.okhistory.org -
#7.Revision und Reenactment - De Gruyter
Revision und Reenactment. In the King of Prussia (1983). From the book Theater als Zeitmaschine. Stefanie Diekmann. https://doi.org/10.14361/transcript. 於 www.degruyter.com -
#8.French and Indian War Reenactment Activities in Lake George ...
Battle reenactments will take place at 2:00 pm on Saturday and Sunday. Drills and skirmishes will take place at 11:00 am on both of those days. The entire ... 於 www.fortwilliamhenry.com -
#9.Neural Rendering and Reenactment of Human Actor Videos
We evaluate our method for the reenactment of another person that is tracked in order to obtain the motion data, and show video results generated from ... 於 vcai.mpi-inf.mpg.de -
#10.Re-enactment Definition & Meaning | Dictionary.com
And Turn looks and feels like some sort of soft-focus dramatic-reenactment segment from a PBS documentary about Aaron Burr. 於 www.dictionary.com -
#11.Reenactment definition and meaning | Collins English Dictionary
Reenactment definition: When a reenactment of a scene or incident takes place, people reenact it. | Meaning, pronunciation, translations and examples. 於 www.collinsdictionary.com -
#12.Battleground Reenactment & Guided Tours | Florida State Parks
... the sights and sounds of history during the first full weekend of March when thousands of visitors attend the annual Natural Bridge battle reenactment. 於 www.floridastateparks.org -
#13.Introduction: What Is Reenactment? - jstor
intellectual enrichment, and sociality, reenactment is booming. History enthusi asts gather weekly to enact past events, television history programs are ... 於 www.jstor.org -
#14.The Boston Tea Party Reenactment | December 16, 1773
Every year on December 16 we celebrate our freedom and the reenactment of the Boston Tea Party. March from the Old South Meeting House To Griffins Wharf. 於 www.bostonteapartyship.com -
#15.Slave Rebellion Reenactment – November 8-9 2019
Slave Rebellion Reenactment is a community-engaged artist performance and film production that, on November 8-9, 2019, reimagined the German Coast Uprising ... 於 www.slave-revolt.com -
#16.The Routledge Handbook of Reenactment Studies 1st Edition
Amazon.com: The Routledge Handbook of Reenactment Studies: 9781032084251: Agnew, Vanessa, Lamb, Jonathan, Tomann, Juliane: Books. 於 www.amazon.com -
#17.reenactment - Dictionary Definition : Vocabulary.com
To enact is to do or make something, and to reenact is to do it again. A reenactment is the action of performing a new version of an old event, usually in a ... 於 www.vocabulary.com -
#18.Real-Time Face Capture and Reenactment of RGB Videos
We present a novel approach for real-time facial reenactment of a monocular target video sequence (e.g., Youtube video). The source sequence is also a ... 於 ieeexplore.ieee.org -
#19.Reenactment Information - FRIENDS OF FORT DAVIDSON
Battle of Pilot Knob 2021 Reenactment is upon us! Friends of Fort Davidson · THE HISTORICAL RECORDS OF THE SEP 25-26, 2021. The Battle of Pilot Knob Returns The ... 於 www.battleofpilotknob.org -
#20.89 Synonyms & Antonyms for REENACTMENT | Thesaurus.com
Find 89 ways to say REENACTMENT, along with antonyms, related words, and example sentences at Thesaurus.com, the world's most trusted free thesaurus. 於 www.thesaurus.com -
#21.Slave Rebellion Reenactment - Dread Scott
Our reenactment restaged and reinterpreted Deslondes' German Coast Uprising of 1811—the largest rebellion of enslaved people in United States history, which ... 於 www.dreadscott.net -
#22.Battle for the Airfield WWII Re-Enactment - The American ...
The American Heritage Museum presents the Battle for the Airfield World War II Re-Enactment, one of the premier events of its type in the United States! 於 www.americanheritagemuseum.org -
#23.Historical Reenactment | SpringerLink
Historical Reenactment. From Realism to the Affective Turn. Editors; (view affiliations). Iain McCalman ... 於 link.springer.com -
#24.reenactment - Chinese translation – Linguee
Many translated example sentences containing "reenactment" – Chinese-English dictionary and search engine for Chinese translations. 於 www.linguee.com -
#25.Toward a virtual reenactment of history: Video games and the ...
With the continuing development of gaming technology, the experience of playing a video game has become increasingly similar to reenactment. A ... 於 www.tandfonline.com -
#26.All periods considered (historical reenactment and its methods)
Historical reenactment is a method of recreating an event or historical period (usually a battle or collective event) by adhering as much as possible to the ... 於 passes-present.eu -
#27.Oxford Handbook of Dance and Reenactment
Starting from differences between reenactment and the more established practice of historical reconstruction, leading practitioners and theorists ask how ... 於 www.oxfordhandbooks.com -
#28.Historical reenactment - Wikipedia
Compagnies Franches de la Marine reenactors in Quebec (2006). Historical reenactment (or re-enactment) is an educational or entertainment activity in which ... 於 en.wikipedia.org -
#29.Revolutionary War reenactment returns to Elmira-area park
The Battle of Newtown, considered a turning point in the American Revolution, will come to life this weekend at Newtown Battlefield State ... 於 www.stargazette.com -
#30.Olustee Reenactment Menu
45th Annual Reenactment of the. Battle of Olustee or Ocean Pond Olustee Battlefield Historic State Park, Florida. February 18-20, 2022. Admission ... 於 battleofolustee.org -
#31.Reenactment | Etsy
Check out our reenactment selection for the very best in unique or custom, handmade pieces from our clothing shops. 於 www.etsy.com -
#32.Performing Remains: Art and War in Times of Theatrical ...
Performing Remains : Art and War in Times of Theatrical Reenactment book cover ... into the aesthetic and political potential of reenactments. 於 www.routledge.com -
#33.Living History - Appomattox Court House National Historical ...
Living history is an important part of our programming here at the park. The park's own staff provides first-person historic character ... 於 www.nps.gov -
#34.Crafting 'The Indian': Knowledge, Desire, And Play In Indianist ...
Knowledge, Desire, and Play in Indianist Reenactment. Petra Tjitske Kalshoven. 272 pages, 17 illus., bibliog., index. ISBN 978-0-85745- ... 於 www.berghahnbooks.com -
#35.Revolutionary War Battle of Camden Reenactment
To find our Reenactment event, the largest in the Southeast, (the largest in the nation in 2020), just follow the SOUNDS OF THE GUNS! You will ... 於 www.cityofcamden.org -
#36.160th Anniversary of the Battle of Perryville Reenactment
160th Anniversary of the Battle of Perryville Reenactment. Perryville Battlefield State Historic Site. P.O. Box 296. 1825 Battlefield Rd. (KY 1920). 於 parks.ky.gov -
#37.reenactment-翻译为中文-例句英语
使用Reverso Context: He included a reenactment of her execution in his 1947 play The Maids.,在英语-中文情境中翻译"reenactment" 於 context.reverso.net -
#38.Reenactments | Moland House
Reenactments. KEEP HISTORY ALIVE IN WARWICK TOWNSHIP! Each year we have a reenactment to celebrate Washington's August 1777 encampment. 於 moland.org -
#39.Camp Wildcat Reenactment back in action after battle site was ...
LONDON, Ky. (WYMT) - The Camp Wildcat Reenactment kicked off Friday, October 15th, and ended Sunday, October 17th. 於 www.wymt.com -
#40.reenactment - Wiktionary
NounEdit · The process of enacting again. the reenactment of a former law · The repetition of an earlier (usually historic) event, as a performance or social ... 於 en.wiktionary.org -
#41.Reenactment - The Free Dictionary
1. To enact again: reenact a law. 2. a. To perform again: reenacted the dancer's movements. b. To act out or re-create dramatically (a historical event, for ... 於 www.thefreedictionary.com -
#42.Gettysburg Reenactment Official Site - Gettysburg Reenactment
Gettysburg Civil War Battle Reenactment in Gettysburg Pennsylvania. 於 www.gettysburgreenactment.com -
#44.Reenactment | Hampshire Cultural Trust
Anglo-Saxon reenactment group Herigeas Hundas will be descending upon the museum with an action packed day of combat displays and living history talks as ... 於 www.hampshireculture.org.uk -
#45.Introduction: What Is Reenactment? - Digital Commons ...
intellectual enrichment, and sociality, reenactment is booming. History enthusi- asts gather weekly to enact past events, television history programs are ... 於 digitalcommons.wayne.edu -
#46.Revolutionary War Reenactment - Cantigny
Join Cantigny and The North West Territory Alliance for a two-day Revolutionary War Reenactment. Get a feel for camp life, experience a battle and enjoy the ... 於 cantigny.org -
#47.Liberty or Death Reenactments - Historic St. John's Church ...
John's Church Foundation has presented historical reenactments of the Second Virginia Convention of March 1775 at its original location. Professional actors ... 於 www.historicstjohnschurch.org -
#48.March 2021 Bentonville Battlefield Reenactment Cancelled
Due to the uncertainty regarding the COVID-19 pandemic, the reenactment, scheduled for March 21-22, 2021 at Bentonville Battlefield has been ... 於 www.ncdcr.gov -
#49.re-enactment noun - Definition, pictures, pronunciation and ...
Definition of re-enactment noun in Oxford Advanced Learner's Dictionary. Meaning, pronunciation, picture, example sentences, grammar, usage notes, ... 於 www.oxfordlearnersdictionaries.com -
#50.reenactment - English-Spanish Dictionary - WordReference.com
reenactment - Translation to Spanish, pronunciation, and forum discussions. 於 www.wordreference.com -
#51.Boston Events | December 16, 1773 Reenactment
Join us each and every year on December 16th in Boston to celebrate and re-enact the single most important event leading up to the American Revolution. 於 www.december16.org -
#52.Repräsentation und Reenactment 978-3-16-160133-0 - Mohr ...
Volker Leppin explores late medieval piety. The key concepts of »representation« and »reenactment« describe God's presence as a given fact bound to certain ... 於 www.mohrsiebeck.com -
#53.Reenactment - Harvard Graduate School of Design
Contemporary types of reenactments challenge the prevalent view that conservation is primarily about an event like a monument, or any object. 於 www.gsd.harvard.edu -
#54.Oath Reenactment Ceremony - Senate.gov
In earlier years, the vice president invited newly sworn senators and their families into his Capitol office for a reenactment for home-state photographers. 於 www.senate.gov -
#55.Revolutionary War Reenacting - Mount Vernon
Our staff member camped with the First Virginia Regiment, who gave insight into their military equipment and why they enjoy reenacting. He… 於 www.mountvernon.org -
#56.Touching the Past: Materializing Time in Traumatic “Living ...
The performers and observers of the 2011 reenactment knew full well that the wooden ... Under certain conditions, modern living history reenactments offer ... 於 www.journals.uchicago.edu -
#57.Documentary - Routledge Handbooks Online
79). Reenactment is a wide-ranging, somewhat nebulous term when applied to nonfiction film, spanning simple and functional reconstructions to the exaggeratedly ... 於 www.routledgehandbooks.com -
#58.Reenactments (2007-10) - Eva & Franco Mattes
Reenactments (2007-10). of historical performances inside videogames, including Marina Abramovic, Gilbert&George, Vito Acconci, Chris Burden and Valie ... 於 0100101110101101.org -
#59.(PDF) Introduction: What Is Reenactment? - ResearchGate
Reenactment thus spans diverse history-themed genres—from theatrical and "living history" performances to museum exhibits, television, film, travelogues, and ... 於 www.researchgate.net -
#60.Reenactment Society - Bristol SU
Reenactment Society. Xfgc. Category. Sports -Team; Recreational; Extreme & Outdoor. Events · Articles. Description. Join This Group. Who are we? Hello! 於 www.bristolsu.org.uk -
#61.#reenactment hashtag on Instagram • Photos and Videos
774k Posts - See Instagram photos and videos from 'reenactment' hashtag. 於 www.instagram.com -
#62.Civil War Reenactment - Union Gap, WA - Central Washington ...
Re-live history at the Civil War Battle Reenactment in Union Gap, WA. Come watch the North and South face off each day! This is Washington State's largest ... 於 www.centralwaagmuseum.org -
#63.Battle of Guilford Courthouse - Greensboro-nc.gov
The Greensboro Parks and Recreation Department sponsors the annual Reenactment of the Battle of Guilford Courthouse at Greensboro Country Park every March. 於 www.greensboro-nc.gov -
#64.Re-enactment of the Battle of Stoney Creek - Hamilton.ca
CANCELLED due to COVID-19 Battlefield House Museum & Park, National Historic Site presents the annual Re-enactment of the Battle of Stoney ... 於 www.hamilton.ca -
#65.Reenactment - Rimini Protokoll
Reenactment. By Stefan Kaegi. 01.01.2016 / Shannon Jackson, "Keywords anthology". Nine-year-old Yaoundé Mulamba Nkita was in French class when he heard his ... 於 www.rimini-protokoll.de -
#66.Reenactment Suite - AI Facial Expressions - D-ID
D-ID's proprietary video facial reenactment technology transforms the way we view faces. The AI-based technology enables the creation of high-quality, ... 於 www.d-id.com -
#67.In Person: Reenactment in Postwar and Contemporary Cinema
In Person: Reenactment in Postwar and Contemporary Cinema delineates a new performative genre based on replay and self-awareness. 於 oxford.universitypressscholarship.com -
#68.reenactment 的中文翻譯| 英漢字典
Reenactment \Re`en*act"ment\ (-?kt"ment), n. The enacting or passing of a law a second time; the renewal of a law. [1913 Webster] 於 cdict.net -
#69.Reenact Definition & Meaning - Merriam-Webster
Definition of reenact · 1 : to enact (something, such as a law) again · 2 : to act or perform again · 3 : to repeat the actions of (an earlier event or incident). 於 www.merriam-webster.com -
#70.Reenactment Information | Cedar Creek Battlefield Foundation
The 158th Battle of Cedar Creek Reenactment October 15th and 16th, 2022. In Commemoration of the 158th Anniversary of the Battle of Cedar Creek, ... 於 www.ccbf.us -
#71.Category:Reenactments - Wikimedia Commons
English: Reenactment is the dressing and behaving (i.e.: reenacting) in the fashion of past historical periods. 於 commons.wikimedia.org -
#72.reenactment - APA Dictionary of Psychology
reenactment n. in some forms of psychotherapy, the process of reliving traumatic events and past experiences and relationships while also reexperiencing the ... 於 dictionary.apa.org -
#73.Reenactments - Newport Historical Society
Reenactments · Weddings in Colonial America · Living History in Downtown Newport · Life During the 1778 Battle of Rhode Island · NHS Initiatives · Helpful Links · Get ... 於 newporthistory.org -
#74.The Reenactment (2021) - IMDb
The Reenactment: Directed by Andrew Ford. With Megan Duffy, Tony Todd, Stephen Wesley Green, Kaitlyn Bausch. A film crew working on a TV show like "Unsolved ... 於 www.imdb.com -
#75.Reenactments: Playing war in the midst of peace | Culture - DW
Whether it's Waterloo or Stalingrad, battle reenactments are booming. In Weimar, things are less bloody: There, the excerpts of ... 於 www.dw.com -
#76.One-shot Face Reenactment Using Appearance Adaptive ...
The paper proposes a novel generative adversarial network for one-shot face reenactment, which can animate a single face image to a different ... 於 arxiv.org -
#77.An embarrassing decision to give this Civil War reenactment ...
Civil War reenactments of this kind minimize the terrible nature of war in general and the terrible legacy of the Civil War on our country ... 於 www.washingtonpost.com -
#78.Military Historical Re-Enactment Club - CAFconnection.ca
If you'd like to try out the medieval combat such as either Heavy Fighting or Fencing however, you'll need to bring your own groin protection (a jock for men, a ... 於 www.cafconnection.ca -
#79.Van Raalte Farm Civil War Muster - Holland, Michigan
The muster and reenactment takes place at VanRaalte Farm Park, which was farmland around the Civil War era and later purchased by the city of Holland in ... 於 www.holland.org -
#80.WWII reenactment this Saturday at Phil Moore Park, rare ...
WWII reenactment this Saturday at Phil Moore Park, rare German tank coming to town ... BOWLING GREEN, Ky. (WBKO) - The 3rd annual Operation Anvil ... 於 www.wbko.com -
#81.Historical Reenactment Groups & Interpreters - Crossroads of ...
1st Battalion, New Jersey Volunteers – 1st Bn NJV. British and Loyalist Reenactment Group. Contact Information: [email protected] ... 於 revolutionarynj.org -
#82.The Siege of Fort Erie Reenactment at Old Fort Erie - Niagara ...
All camp and battle reenactments at Old Fort Erie are free to the public and begin at 10 a.m. at the Welcome Centre. Admission to Old Fort Erie and the Saturday ... 於 www.niagaraparks.com -
#83.Introduction to Reenactments and Reenactors, Part 1 | EXARC
Reenactments, meaning special events that use outside costumed interpreters, ... Planning and executing a reenactment can be a daunting challenge for a site ... 於 exarc.net -
#84.Reenactment - an overview | ScienceDirect Topics
A reenactment is a process in which the participants mimic the actions involved in a specific event or series of events. Instead of being a free-form and ... 於 www.sciencedirect.com -
#85.Reenactments -- Historic Courtroom Theater
Reenactments -- Historic Courtroom Theater ... Historic court cases can provide a window into the past of interest not only to attorneys but to all students of ... 於 justiceforall.ca2.uscourts.gov -
#86.重演紀錄片Reenactment - 認識電影
重演紀錄片Reenactment. 《日曜日式散步者》Le Moulin 黃亞歷,2015. 紀錄片使用「重演」主要是透過戲劇性演出,讓過去的事件「再發生一次」。採取重演的手法很可能是 ... 於 edumovie.culture.tw -
#87.RE-ENACTMENT (noun) definition and synonyms - Macmillan ...
re-enactment Definitions and Synonyms. noun. or reenactment. DEFINITIONS1. 1. the act of recreating a past or historical event. Civil War re-enactments ... 於 www.macmillandictionary.com -
#88.The Reenactment - Va Museum of the Civil War - Virginia ...
While our first event was in May of 1914, our biggest was in September of 1923 between VMI Cadets and United States Marines. At the reenactment, you will ... 於 www.vmi.edu -
#89.157th Anniversary Battle of Pilot Knob Reenactment - Missouri ...
Battle of Pilot Knob State Historic Site will host the 157th anniversary reenactment of the historic Civil War Battle that took place at the site in ... 於 mostateparks.com -
#90.Reenactment Groups & Gunfight Reenactments - Tombstone ...
Tombstone AZ has many choices activities and sightseeing. These businesses represent members of the Tombstone Chamber of Commerce. 於 tombstonechamber.com -
#91.effects of reenactment on toddlers' event memory - PubMed
Four experiments examined (1) whether reenactment improves 18-month-olds' event memory, (2) how effects of reenactment are affected by the time at which the ... 於 pubmed.ncbi.nlm.nih.gov -
#92.reenactment - USS Alabama Battleship Memorial Park
Tag Archives: reenactment ... as the living history crews of the USS ALABAMA, USS DRUM and our visiting crews come together for a day of WWII reenactments. 於 www.ussalabama.com -
#94.Sudden Unexplained Infant Death Investigation, Chapter 7
This chapter details the rationale behind doll reenactments and outlines a performance procedure to follow. CHAPTER 7 CONDUCTING THE DOLL REENACTMENT. Page 3 ... 於 www.cdc.gov -
#95.Reenactment - Hurricane Shoals Park
Annual Civil War Reenactment. This year's reenactments for Art in The Park 2021 will be of two local battles in our area on Northeast Georgia. 於 www.hurricaneshoalspark.org